The Future of Renewable Energy: AI and Data Science in Action
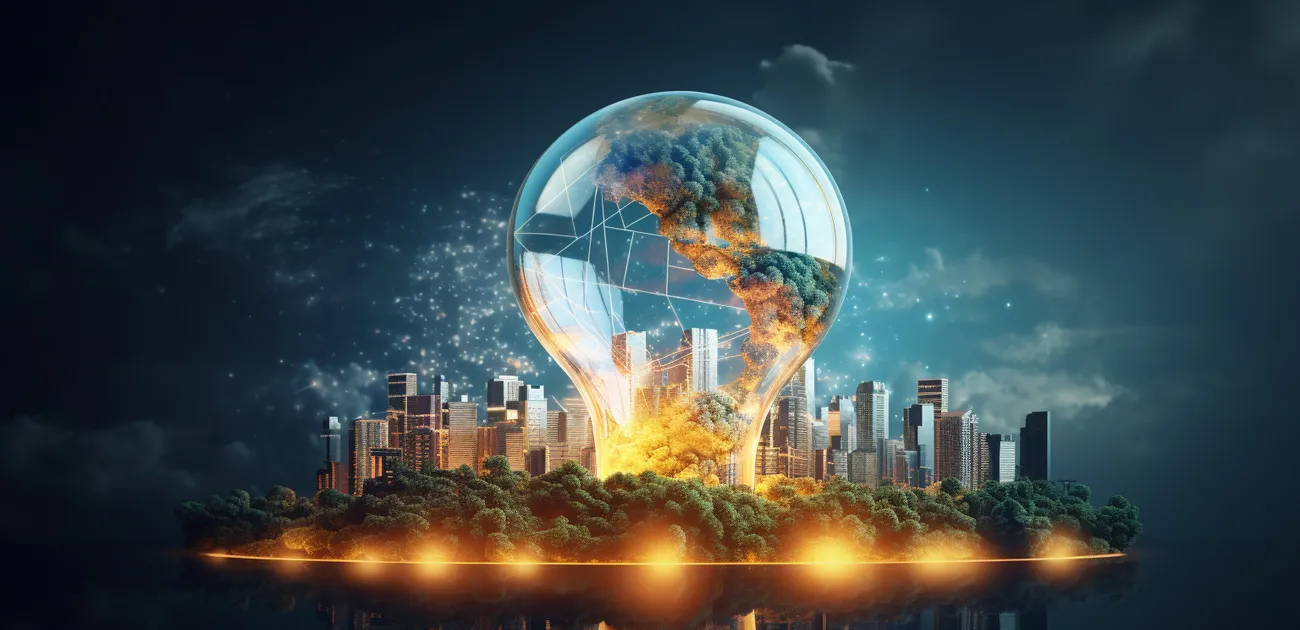
The Future of Renewable Energy: AI and Data Science in Action
Table of Contents
- Introduction
- The Role of AI in Renewable Energy
- Applications of AI in Renewable Energy
- Data Science for Renewable Energy
- Challenges and Opportunities
- Conclusion
Introduction
The global shift towards renewable energy sources has gained significant momentum in recent years, driven by the need to reduce greenhouse gas emissions and mitigate the effects of climate change. As the world transitions away from fossil fuels, new technologies are emerging to accelerate the adoption and efficiency of renewable energy systems. Among these technologies, artificial intelligence (AI) and data science are playing crucial roles in shaping the future of renewable energy.
The Role of AI in Renewable Energy
AI offers immense potential in the renewable energy sector, revolutionizing various aspects of energy generation, storage, management, and optimization. By leveraging machine learning algorithms and advanced analytics, AI can process vast amounts of data, identify patterns, and make intelligent decisions in real-time. This enables renewable energy systems to operate more efficiently, adapt to dynamic conditions, and enhance overall performance.
Applications of AI in Renewable Energy
Optimizing Energy Generation
AI enables the optimization of renewable energy generation by analyzing data from various sources, such as weather forecasts, energy consumption patterns, and grid demand. By incorporating these factors, AI algorithms can dynamically adjust renewable energy generation to match the demand, reducing waste and maximizing system efficiency. For example, AI can optimize the output of solar photovoltaic (PV) systems based on real-time weather conditions, ensuring maximum power generation.
Improving Energy Storage
Energy storage is a critical component of renewable energy systems, enabling the utilization of generated energy during periods of low production. AI can enhance energy storage by optimizing charging and discharging patterns based on electricity prices, demand fluctuations, and weather forecasts. By utilizing machine learning algorithms, energy storage systems can make intelligent decisions to ensure efficient energy utilization, cost-effectiveness, and grid stability.
Enhancing Grid Management
AI plays a pivotal role in managing the complex and decentralized nature of renewable energy integration into the grid. By analyzing real-time data from distributed energy resources (DERs) such as solar and wind farms, AI algorithms can optimize power flow, voltage regulation, and grid stability. AI can also facilitate demand response programs, enabling consumers to adjust their energy consumption based on grid conditions, reducing peak demand and enhancing overall grid efficiency.
Streamlining Maintenance and Operations
Data-driven AI solutions can streamline the maintenance and operations of renewable energy systems, minimizing downtime and optimizing performance. By analyzing sensor data and historical maintenance records, AI algorithms can predict equipment failures, recommend preventive maintenance, and optimize repair schedules. This predictive maintenance approach improves system reliability, reduces costs, and increases the lifespan of renewable energy assets.
Data Science for Renewable Energy
Data science plays a crucial role in harnessing the power of data generated by renewable energy systems. Through advanced data collection, analysis, and modeling techniques, data science enables better decision-making, resource assessment, and energy efficiency.
Data Collection and Analysis
Data science techniques facilitate the collection and analysis of vast amounts of data from renewable energy sources. This includes weather data, power generation data, sensor readings, and grid information. By integrating data from multiple sources, data scientists can gain insights into energy generation patterns, identify potential inefficiencies, and optimize system performance.
Predictive Modeling and Forecasting
Data science enables the development of predictive models and forecasting algorithms for renewable energy systems. By analyzing historical data and considering various factors such as weather conditions, energy demand, and system parameters, data scientists can accurately forecast power generation, demand fluctuations, and energy prices. These forecasts enable proactive decision-making, efficient resource allocation, and grid stability.
Resource Assessment and Site Selection
Data science techniques assist in resource assessment and site selection for renewable energy projects. By analyzing geographical and environmental data, such as wind speeds, solar radiation, and topography, data scientists can identify optimal locations for wind farms, solar installations, and hydroelectric plants. This ensures efficient utilization of resources and maximizes energy production potential.
Demand Response and Energy Efficiency
Data science enables the implementation of demand response programs and energy efficiency measures. By analyzing energy consumption patterns and customer behavior, data scientists can identify opportunities for demand management, load shifting, and energy conservation. These insights help utilities and consumers make informed decisions, reduce energy waste, and promote a sustainable energy ecosystem.
Challenges and Opportunities
While AI and data science offer significant opportunities for the future of renewable energy, several challenges must be addressed to realize their full potential.
Data Accessibility and Quality
Access to reliable and comprehensive data is crucial for AI and data science applications in renewable energy. Ensuring data availability, interoperability, and standardization across different platforms and systems is essential. Additionally, data quality and accuracy play a vital role in the performance and reliability of AI algorithms, highlighting the need for robust data validation and cleansing processes.
Ethical Considerations
As AI and data science become increasingly integrated into renewable energy systems, ethical considerations must be prioritized. Ensuring transparency, fairness, and accountability in AI decision-making processes is essential. Ethical frameworks and guidelines should be developed to address concerns related to data privacy, algorithm bias, and potential social implications.
Interdisciplinary Collaboration
The future of renewable energy heavily relies on interdisciplinary collaboration between experts in AI, data science, renewable energy, policy, and engineering. Collaborative efforts are necessary to develop innovative solutions, address complex challenges, and foster knowledge sharing. Public-private partnerships and academic-industry collaborations can accelerate progress and facilitate the integration of AI and data science in renewable energy systems.
Policy and Regulatory Frameworks
Establishing supportive policy and regulatory frameworks is crucial for the widespread adoption and integration of AI and data science in renewable energy. Clear guidelines regarding data ownership, privacy, and cybersecurity should be established. Additionally, incentives and funding mechanisms can encourage research, development, and deployment of AI-driven renewable energy technologies.
Conclusion
The future of renewable energy is intrinsically linked to the advancements in AI and data science. These technologies offer unprecedented opportunities to optimize energy generation, storage, grid management, and operational efficiency. By leveraging the power of data and intelligent algorithms, renewable energy systems can become more reliable, cost-effective, and sustainable. However, addressing challenges related to data accessibility, ethics, collaboration, and policy frameworks is essential for realizing the full potential of AI and data science in shaping the future of renewable energy.