Overcoming Challenges in the Renewable Energy Industry with Data Science
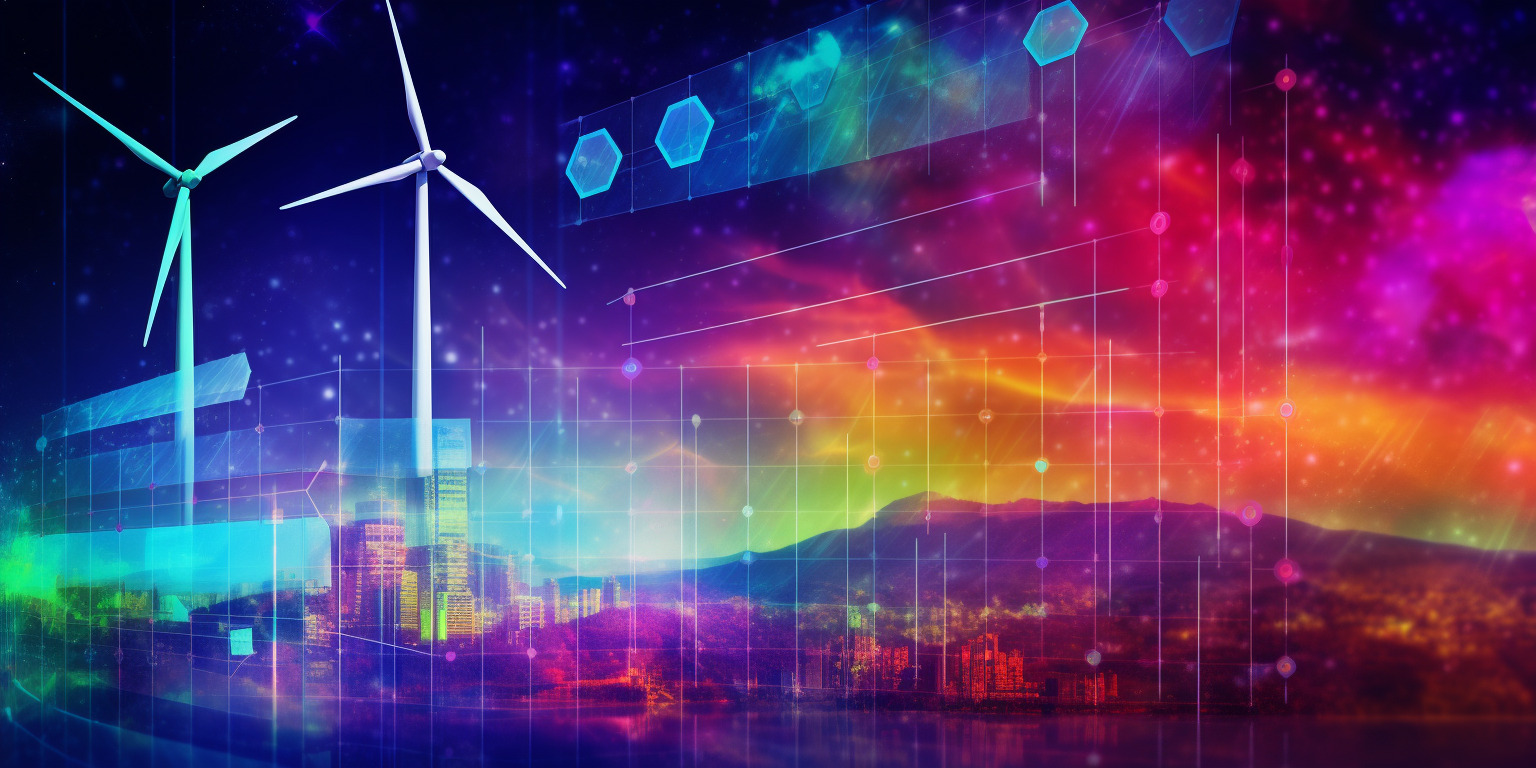
Overcoming Challenges in the Renewable Energy Industry with Data Science
Table of Contents
- Introduction
- The Role of Data Science in the Renewable Energy Industry
- Challenges in the Renewable Energy Industry
- How Data Science Can Address these Challenges
- Conclusion
Introduction
Renewable energy sources such as solar, wind, and hydroelectric power play a crucial role in addressing the global challenges of climate change and sustainable development. However, the renewable energy industry faces several significant challenges that can hinder its growth and widespread adoption. In recent years, data science has emerged as a powerful tool to overcome these challenges and drive innovation in the renewable energy sector. This blog post explores the role of data science in the industry and how it can help overcome key challenges.
The Role of Data Science in the Renewable Energy Industry
Data science involves the collection, analysis, and interpretation of large volumes of data to extract meaningful insights and make informed decisions. In the renewable energy industry, data science can play a transformative role by leveraging advanced analytics techniques and machine learning algorithms to optimize renewable energy generation, improve resource allocation, enhance grid integration, and enable predictive maintenance of renewable energy assets.
Challenges in the Renewable Energy Industry
Intermittency of Renewable Energy Sources
One of the primary challenges in the renewable energy industry is the intermittency of renewable energy sources. Unlike traditional fossil fuel-based power plants that provide a consistent supply of electricity, renewable energy generation fluctuates based on weather conditions and other factors. This intermittency poses challenges for grid operators and energy system planners, as they need to ensure a stable and reliable power supply.
Optimal Resource Allocation
Another challenge is the optimal allocation of renewable energy resources. The location, size, and type of renewable energy installations significantly impact their efficiency and effectiveness. Data science can help analyze various factors such as solar irradiation, wind patterns, geographical terrain, and demand patterns to determine the optimal placement and sizing of renewable energy installations. By optimizing resource allocation, data science can improve energy production and reduce costs.
Grid Integration and Stability
Integrating renewable energy sources into existing power grids poses technical and operational challenges. The intermittent nature of renewables and their decentralized nature require innovative solutions for grid integration and stability. Data science techniques such as machine learning can be applied to predict renewable energy generation and optimize grid operations accordingly. By effectively managing the integration of renewable energy sources, data science can enhance grid stability and reliability.
Predictive Maintenance
Maintaining renewable energy assets is essential for ensuring their long-term performance and minimizing downtime. Traditional maintenance approaches based on fixed schedules can be inefficient and costly. Data science enables predictive maintenance by analyzing sensor data, historical performance data, and environmental factors to identify patterns and predict maintenance needs. This proactive approach helps optimize maintenance schedules, reduce costs, and maximize energy generation.
How Data Science Can Address these Challenges
Forecasting and Predictive Analytics
Data science techniques such as time series analysis, regression models, and machine learning algorithms can be used for accurate forecasting of renewable energy generation. By analyzing historical weather data, energy production data, and other relevant factors, data scientists can develop predictive models that forecast renewable energy generation with high precision. These forecasts enable grid operators to plan and optimize power distribution, reducing the impact of intermittency.
Optimization Algorithms
Optimization algorithms play a crucial role in determining the optimal placement, sizing, and operation of renewable energy installations. Data science techniques, including linear programming, genetic algorithms, and mixed-integer programming, can optimize resource allocation based on multiple factors, such as energy demand, geographical constraints, and cost considerations. By leveraging these optimization algorithms, data scientists can maximize energy production while minimizing costs and environmental impact.
Machine Learning for Grid Integration
Machine learning algorithms can analyze historical data from renewable energy sources, weather patterns, and grid operations to identify patterns and optimize grid integration. By learning from past experiences, machine learning models can predict renewable energy generation, forecast electricity demand, and dynamically adjust grid parameters to ensure stability and reliability. These data-driven insights enable grid operators to effectively manage the integration of renewable energy sources into the existing power grid.
Condition Monitoring and Predictive Maintenance
Data science techniques such as anomaly detection, pattern recognition, and machine learning algorithms can be used for condition monitoring and predictive maintenance of renewable energy assets. By continuously monitoring sensor data, data scientists can detect anomalies and potential failures in real-time. This allows for timely maintenance interventions, minimizing downtime, and optimizing the performance and lifespan of renewable energy assets.
Conclusion
The renewable energy industry faces various challenges that can hinder its growth and integration into the existing energy infrastructure. However, data science offers promising solutions to overcome these challenges. By leveraging advanced analytics techniques, optimization algorithms, and machine learning models, data scientists can optimize renewable energy generation, improve resource allocation, enhance grid integration, and enable predictive maintenance. As the renewable energy sector continues to expand, the role of data science will become increasingly vital in driving innovation and ensuring a sustainable and clean energy future.