Optimizing Wind Farm Performance through Advanced Data Analytics
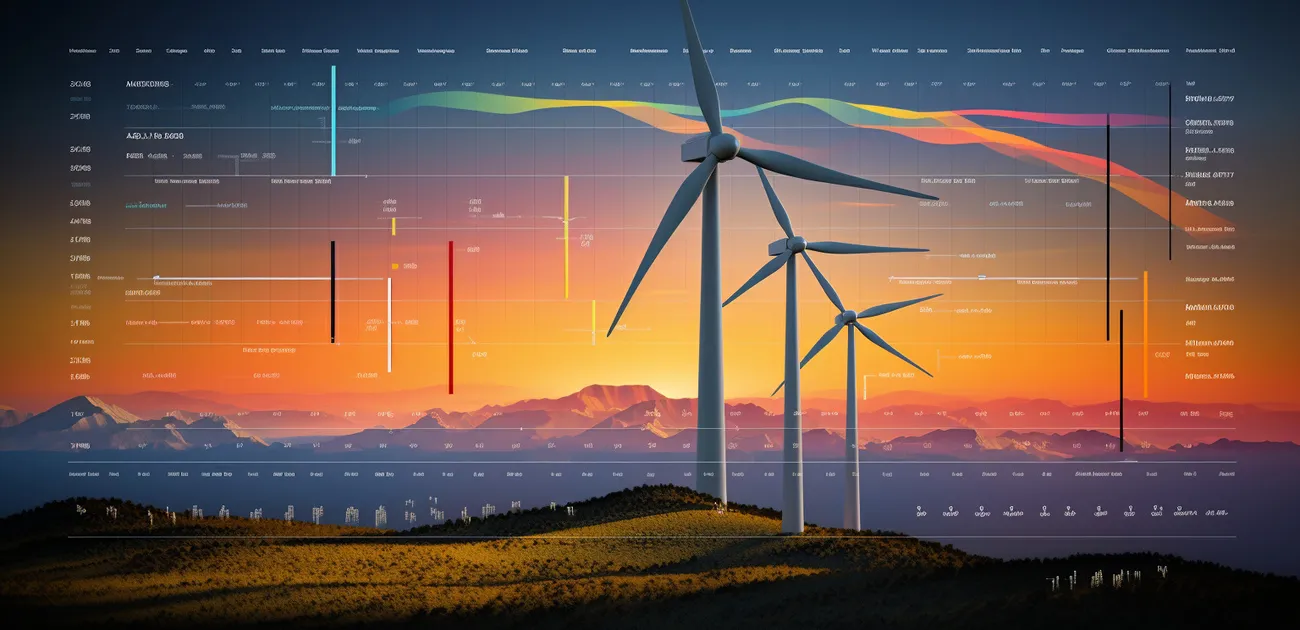
Optimizing Wind Farm Performance through Advanced Data Analytics
Table of Contents
- Introduction
- Understanding Wind Farm Performance
- Importance of Data Analytics
- Data Collection and Monitoring
- Data Cleaning and Preprocessing
- Predictive Modeling and Forecasting
- Maintenance Optimization
- Power Curve Analysis
- Performance Benchmarking
- Conclusion
Introduction
Wind energy has emerged as a significant source of renewable power generation. Wind farms, consisting of multiple wind turbines, play a vital role in harnessing wind energy. However, optimizing the performance of wind farms is crucial to maximize energy production and ensure operational efficiency. In this blog post, we will explore how advanced data analytics can be leveraged to optimize wind farm performance.Understanding Wind Farm Performance
Before delving into data analytics, it's essential to understand the factors that impact wind farm performance. Several variables, such as wind speed, wind direction, turbine characteristics, and environmental conditions, influence the energy output of wind turbines. By analyzing these factors, we can identify opportunities for improvement.Importance of Data Analytics
Data analytics plays a vital role in unlocking the full potential of wind farms. By analyzing large volumes of data collected from various sources, we can gain valuable insights into turbine behavior, operational patterns, and performance trends. These insights enable us to optimize operations, reduce downtime, improve maintenance planning, and increase energy production.Data Collection and Monitoring
Accurate and reliable data collection is the foundation of effective wind farm performance optimization. Wind turbines are equipped with sensors that capture real-time data on parameters like wind speed, power output, temperature, and vibration. Additionally, meteorological stations in and around the wind farm provide crucial weather data. All this information is collected and stored in a centralized database for further analysis.Data Cleaning and Preprocessing
Raw data collected from wind turbines and meteorological stations often contains noise, outliers, and missing values. Data cleaning and preprocessing techniques are employed to address these issues. This involves removing or correcting erroneous data points, handling missing values through interpolation or imputation, and normalizing the data for uniformity.Predictive Modeling and Forecasting
Predictive modeling techniques, such as [machine learning algorithms](innovations-in-wind-farm-operations-through-machine-learning), can be applied to historical data to build models that predict future wind farm performance. These models take into account factors like wind speed, wind direction, and turbine characteristics to forecast energy production and identify potential underperforming turbines. By proactively addressing these issues, operators can optimize performance and prevent potential losses.Maintenance Optimization
Scheduled maintenance is crucial for wind farm longevity and efficiency. Data analytics helps optimize maintenance practices by identifying patterns and trends in equipment performance. By analyzing historical maintenance records and correlating them with performance data, operators can determine optimal maintenance schedules, reduce downtime, and minimize maintenance costs.Power Curve Analysis
Power curves depict the relationship between wind speed and turbine power output. Analyzing power curves allows operators to assess turbine performance and detect deviations from expected behavior. By comparing actual power curves with manufacturer specifications, operators can identify and rectify performance issues, such as turbine misalignment or faulty sensors.Performance Benchmarking
Benchmarking wind farm performance against industry standards and best practices provides valuable insights into operational efficiency. Data analytics facilitates performance benchmarking by comparing key performance indicators (KPIs) across different wind farms and identifying areas of improvement. This enables operators to learn from top-performing wind farms and implement strategies to enhance their own performance.Conclusion
Optimizing wind farm performance through advanced data analytics is a powerful approach to maximize energy production, improve operational efficiency, and reduce costs. By leveraging data collection, cleaning, predictive modeling, maintenance optimization, power curve analysis, and performance benchmarking, operators can gain valuable insights and make data-driven decisions to optimize their wind farm's performance. With the increasing adoption of data analytics in the renewable energy sector, we can expect significant advancements in wind farm performance optimization in the coming years.Remember, wind farm performance optimization is an ongoing process. Regular data analysis and continuous improvement efforts will ensure long-term success and sustainability in the wind energy industry.