The Role of Machine Learning in Maximizing Energy Yield from Renewable Sources
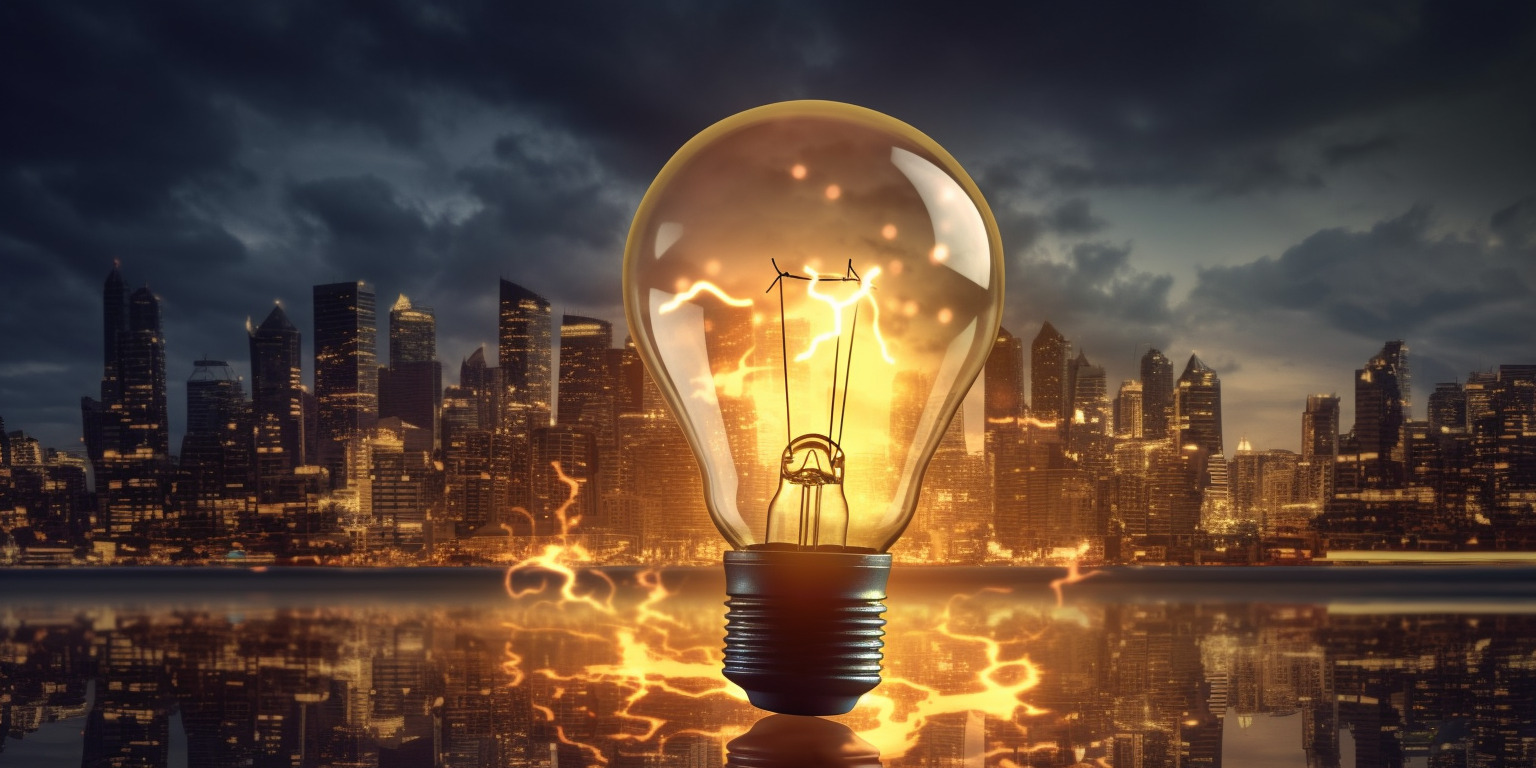
The Role of Machine Learning in Maximizing Energy Yield from Renewable Sources
Table of Contents
- Introduction
- Understanding Renewable Energy
- Challenges in Maximizing Energy Yield
- How Machine Learning Enhances Energy Yield
- Applications of Machine Learning in Renewable Energy
- Case Studies
- Future Possibilities and Limitations
- Conclusion
Introduction
Renewable energy sources such as solar, wind, and hydro power play a crucial role in addressing the global energy crisis and mitigating the effects of climate change. However, maximizing the energy yield from these sources can be challenging due to various factors such as weather variability, intermittent generation patterns, and complex system dynamics. In recent years, machine learning (ML) has emerged as a powerful tool for optimizing renewable energy systems and improving energy production efficiency. This blog post explores the role of machine learning in maximizing energy yield from renewable sources and highlights its potential applications and benefits.
Understanding Renewable Energy
Renewable energy refers to energy obtained from sources that are naturally replenished, such as sunlight, wind, and flowing water. Unlike fossil fuels, which are finite and contribute to environmental pollution, renewable energy sources provide a clean and sustainable alternative. However, harnessing renewable energy efficiently requires advanced technologies and optimization strategies.
Challenges in Maximizing Energy Yield
Maximizing energy yield from renewable sources is not a straightforward task. It involves addressing several challenges, including:
-
Weather Variability: Renewable energy generation is heavily influenced by weather conditions. Accurate forecasting of solar irradiance, wind speed, and hydrological patterns is crucial for optimizing energy production.
-
Intermittency: Unlike traditional power plants, renewable energy sources exhibit intermittent generation patterns. This intermittency makes it challenging to match energy supply with demand consistently.
-
Complex System Dynamics: Renewable energy systems often involve complex interactions between various components such as solar panels, wind turbines, and hydroelectric generators. Optimizing the performance of these systems requires understanding and modeling their intricate dynamics.
How Machine Learning Enhances Energy Yield
Machine learning techniques offer valuable tools for addressing the challenges in maximizing energy yield from renewable sources. By leveraging large datasets and powerful algorithms, ML can provide insights and predictive capabilities that enable smarter decision-making and optimization. Some ways in which machine learning enhances energy yield include:
-
Data-driven Forecasting: Machine learning algorithms can analyze historical weather data and other relevant variables to forecast energy generation patterns accurately. These forecasts enable proactive scheduling of energy supply and demand, improving the overall energy yield.
-
System Optimization: ML models can optimize the operation and control of renewable energy systems by adapting to real-time conditions. By continuously learning and adjusting system parameters, ML algorithms can maximize energy production and minimize losses.
-
Anomaly Detection: Machine learning can detect and diagnose anomalies in renewable energy systems, such as faulty solar panels or underperforming wind turbines. Early identification of such issues allows for timely maintenance and ensures optimal energy yield.
Applications of Machine Learning in Renewable Energy
1. Solar Power
Machine learning techniques have been extensively used in solar power systems for various applications, including:
- Solar irradiance prediction for accurate energy forecasting.
- Fault detection and diagnostics of solar panels.
- Optimization of solar panel orientation and tilt angles.
- Real-time control of solar tracking systems.
2. Wind Power
In wind power systems, machine learning has proven beneficial in the following areas:
- Wind speed and power output prediction.
- Fault detection and predictive maintenance of wind turbines.
- Optimization of turbine operation based on weather conditions.
- Wind farm layout optimization for increased energy production.
3. Hydro Power
Machine learning techniques can also enhance hydro power systems by:
- Accurate streamflow forecasting for optimal reservoir management.
- Real-time control of hydroelectric generators based on energy demand.
- Early detection of equipment failures or leaks in hydroelectric plants.
- Optimization of water flow scheduling for maximum energy generation.
Case Studies
1. Solar Power Plant Optimization
In a case study conducted at a solar power plant, machine learning algorithms were employed to optimize the plant's energy production. By analyzing historical weather data and system parameters, the ML model predicted solar irradiance and adjusted the orientation of solar panels in real-time. This optimization approach resulted in a significant increase in energy yield and improved overall plant performance.
2. Wind Turbine Performance Prediction
In another case study, machine learning was utilized to predict the performance of wind turbines. By training ML models on historical wind speed data and turbine sensor readings, it became possible to identify potential issues and predict turbine failures in advance. This proactive maintenance approach reduced downtime and increased the energy yield of the wind farm.
Future Possibilities and Limitations
The application of machine learning in maximizing energy yield from renewable sources holds immense potential. However, certain limitations and challenges need to be considered:
-
Data Availability: Machine learning models heavily rely on large and diverse datasets. Access to quality data from renewable energy systems can be a limitation, especially in remote locations or developing regions.
-
Model Interpretability: As machine learning algorithms become more complex, interpreting their decisions and recommendations becomes challenging. Explainable AI techniques need to be developed to ensure transparency and trust in the decision-making process.
-
Scalability: Scaling machine learning solutions to larger renewable energy systems and grids requires significant computational resources and infrastructure. Overcoming scalability challenges is essential for widespread adoption.
Conclusion
Machine learning is revolutionizing the renewable energy sector by maximizing energy yield from renewable sources. By leveraging advanced algorithms and data-driven insights, ML enables accurate forecasting, system optimization, and anomaly detection. From solar power to wind and hydroelectric systems, machine learning finds applications across various renewable energy domains. While challenges exist, the future holds immense possibilities for using machine learning to create a sustainable and efficient energy landscape.