Financial Forecasting for Renewable Energy Projects: A Data-driven Approach
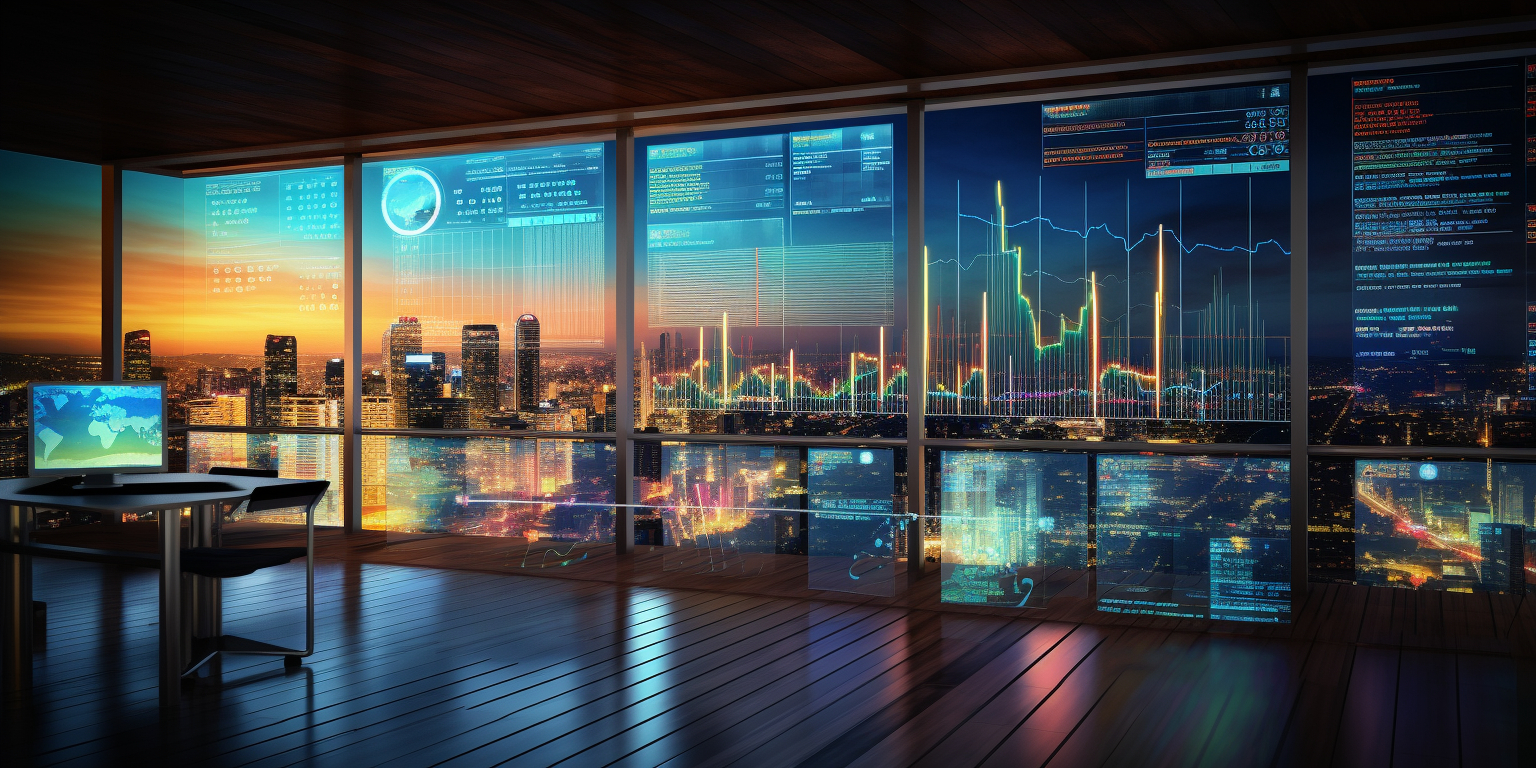
Financial Forecasting for Renewable Energy Projects: A Data-driven Approach
Table of Contents
- Introduction
- Importance of Financial Forecasting
- Data-driven Approach
- Key Data Points for Financial Forecasting
- Techniques for Financial Forecasting
- Challenges and Mitigation
- Conclusion
Introduction
In today's rapidly evolving energy landscape, renewable energy projects play a vital role in meeting sustainability goals. However, these projects require accurate financial forecasting to ensure their viability and long-term success. This blog post explores the significance of financial forecasting for renewable energy projects and highlights the benefits of employing a data-driven approach.Importance of Financial Forecasting
Financial forecasting serves as a roadmap for renewable energy projects by estimating potential revenues, costs, and profitability over a specified period. Accurate forecasting allows project developers, investors, and stakeholders to make informed decisions and assess the project's feasibility. By providing a clear picture of the project's financial performance, forecasting helps secure financing, attracts investors, and maximizes return on investment.Data-driven Approach
A data-driven approach to financial forecasting leverages historical and real-time data to generate insights and make reliable projections. With the availability of vast amounts of data from various sources, such as weather patterns, energy production, market trends, and regulatory policies, harnessing this data can significantly enhance the accuracy of forecasts. Data-driven models enable project teams to analyze complex interdependencies, identify trends, and make more informed decisions.Key Data Points for Financial Forecasting
To develop an accurate financial forecast for renewable energy projects, several key data points must be considered:- Resource Data: Data related to solar radiation, wind speeds, hydrological patterns, or any other relevant renewable resource is crucial for predicting energy generation potential.
- System Performance Data: Historical data on energy production and performance metrics of the renewable energy system, including factors like availability, reliability, and downtime.
- Market Data: Information on energy market dynamics, such as electricity prices, incentives, subsidies, and regulatory policies, helps estimate revenue streams and project profitability.
- Cost Data: Detailed cost data for project development, installation, operation, and maintenance, including equipment, labor, financing, insurance, and decommissioning costs.
- Financial Data: Historical financial data, including capital expenditure, operational expenses, revenue, taxes, and cash flow, enables the evaluation of project profitability and financial viability.
Techniques for Financial Forecasting
Several techniques can be employed for financial forecasting in renewable energy projects, including:Time Series Analysis
Time series analysis utilizes historical data to identify patterns, trends, and seasonality. By analyzing past energy production and market data, this method can predict future performance based on recurring patterns. Time series models, such as ARIMA (AutoRegressive Integrated Moving Average), can capture complex dependencies and assist in forecasting energy generation and revenue.Monte Carlo Simulation
Monte Carlo simulation involves running multiple iterations using probabilistic models to generate a range of possible outcomes. By considering uncertainties and random variables, this method provides a probabilistic assessment of project profitability. Monte Carlo simulation can incorporate various factors, such as resource variability, equipment failure rates, and market price fluctuations, to assess financial risks and optimize project planning.Sensitivity Analysis
Sensitivity analysis explores the impact of changes in key parameters on project outcomes. By varying variables like energy prices, project costs, or financing terms, it quantifies the sensitivity of financial metrics such as net present value (NPV) and internal rate of return (IRR). This technique helps identify the most influential factors affecting project profitability and guides decision-making processes.Challenges and Mitigation
Financial forecasting for renewable energy projects comes with its own set of challenges, including data availability, accuracy, and uncertainties surrounding market dynamics. However, these challenges can be mitigated through strategies such as:-
Ensuring data quality through rigorous validation and verification processes.
-
Incorporating a range of scenarios and sensitivity analysis to account for uncertainties.
-
Collaborating with domain experts, data scientists, and financial professionals to develop robust forecasting models.
-
Regularly updating and refining the forecasting models as new data and market insights become available.