Anticipating Equipment Failures in Renewable Energy Infrastructure with Predictive Maintenance
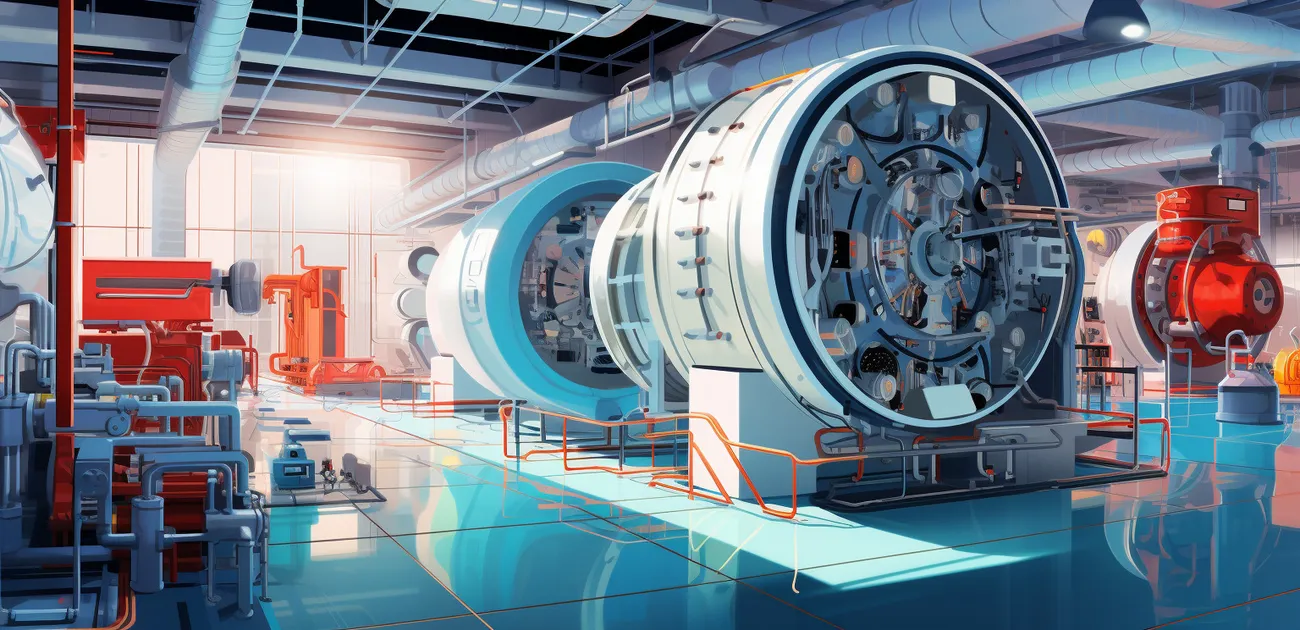
Anticipating Equipment Failures in Renewable Energy Infrastructure with Predictive Maintenance
Table of Contents
- Introduction
- Understanding Predictive Maintenance
- Challenges in Renewable Energy Infrastructure
- Benefits of Predictive Maintenance
- Implementing Predictive Maintenance in Renewable Energy
- Data Collection and Analysis
- Machine Learning Models for Predictive Maintenance
- Real-World Examples
- Conclusion
Introduction
Renewable energy infrastructure, such as wind turbines and solar panels, plays a vital role in our transition to a sustainable future. However, these complex systems are prone to equipment failures, which can result in costly downtime and decreased energy production. To mitigate these risks, predictive maintenance has emerged as a powerful approach to anticipate and prevent equipment failures. In this blog post, we will delve into the world of predictive maintenance and explore how it can revolutionize the maintenance practices in renewable energy infrastructure.
Understanding Predictive Maintenance
Predictive maintenance is a proactive maintenance strategy that leverages data analysis, machine learning, and statistical models to predict equipment failures before they occur. By monitoring various parameters and patterns, predictive maintenance algorithms can identify early warning signs and trigger maintenance actions to prevent catastrophic failures. Unlike traditional preventive maintenance, which relies on fixed schedules, predictive maintenance allows for more efficient and targeted maintenance activities, saving time and resources.
Challenges in Renewable Energy Infrastructure
Renewable energy infrastructure presents unique challenges for maintenance teams due to its remote locations, vast size, and intricate components. These challenges include:
-
Data Availability: Collecting comprehensive and high-quality data from renewable energy assets can be challenging. Remote locations and limited connectivity make it difficult to obtain real-time data on equipment health and performance.
-
Complex Equipment: Renewable energy infrastructure consists of complex equipment with interdependencies. Traditional rule-based systems may not effectively capture the intricacies of these systems, necessitating advanced data-driven techniques.
-
Harsh Environmental Conditions: Wind turbines and solar panels are exposed to harsh environmental conditions such as extreme temperatures, humidity, and corrosive elements. These conditions accelerate equipment degradation, making early detection of failures crucial.
Benefits of Predictive Maintenance
Implementing predictive maintenance in renewable energy infrastructure offers several notable benefits:
-
Reduced Downtime: By identifying potential failures in advance, maintenance activities can be scheduled proactively, minimizing downtime and ensuring continuous energy production.
-
Improved Equipment Lifespan: Predictive maintenance allows for early detection of equipment degradation, enabling timely repairs or replacements. This extends the lifespan of assets, reducing capital expenditures.
-
Optimized Maintenance Planning: Predictive maintenance provides insights into the health and performance of individual components, enabling optimized maintenance planning based on actual conditions rather than fixed schedules.
-
Cost Savings: By preventing catastrophic failures and optimizing maintenance activities, predictive maintenance reduces overall maintenance costs, including emergency repairs and unplanned downtime.
Implementing Predictive Maintenance in Renewable Energy
To implement predictive maintenance effectively in renewable energy infrastructure, the following steps can be taken:
-
Data Collection and Integration: Collecting relevant data from various sources, including sensors, SCADA systems, and historical maintenance records, is the first step. Integration of data into a centralized platform ensures accessibility and enables comprehensive analysis.
-
Data Preprocessing and Feature Engineering: Data preprocessing involves cleaning, aggregating, and transforming raw data into a suitable format for analysis. Feature engineering focuses on extracting meaningful features that capture equipment health and performance characteristics.
-
Machine Learning Models for Predictive Maintenance: Utilize machine learning algorithms such as supervised learning (e.g., classification, regression), unsupervised learning (e.g., clustering), and time series analysis to develop predictive maintenance models. These models can learn patterns and anomalies from historical data and predict failures based on real-time inputs.
-
Integration with Maintenance Workflow: Predictive maintenance outputs should be integrated into the existing maintenance workflow to trigger proactive maintenance actions. This may involve generating work orders, scheduling repairs, and updating asset management systems.
Data Collection and Analysis
Accurate and comprehensive data collection is crucial for the success of predictive maintenance initiatives. It involves monitoring equipment health parameters such as temperature, vibration, current, and voltage. Advanced sensors and IoT devices can be deployed to capture real-time data. Additionally, historical maintenance records and operational data should be included for training machine learning models. Analyzing this data helps identify patterns, correlations, and anomalies that can serve as indicators of potential failures.
Machine Learning Models for Predictive Maintenance
Various machine learning models can be employed for predictive maintenance in renewable energy infrastructure:
-
Regression Models: Regression models such as linear regression, decision trees, and random forests can be used to predict equipment degradation and estimate remaining useful life (RUL). These models utilize historical data and equipment health parameters to forecast future conditions.
-
Classification Models: Classification models such as logistic regression, support vector machines (SVM), and neural networks can be applied to predict failure events or the probability of failure within a specified timeframe. These models can assist in scheduling maintenance activities accordingly.
-
Anomaly Detection Models: Anomaly detection models, such as autoencoders and one-class SVM, can identify abnormal behavior or outliers in equipment data. By flagging unusual patterns, these models enable the detection of potential failures or performance deviations.
Real-World Examples
Several real-world examples demonstrate the effectiveness of predictive maintenance in renewable energy infrastructure:
-
Wind Turbine Monitoring: Predictive maintenance systems have been deployed in wind farms to monitor turbine health parameters, predict failures, and optimize maintenance schedules. By identifying early signs of component wear, these systems have reduced downtime and maintenance costs.
-
Solar Panel Diagnostics: Machine learning algorithms have been employed to analyze solar panel performance data and detect deviations from expected power output. By identifying underperforming panels or potential faults, maintenance teams can take proactive measures to maximize energy production.
-
Hydropower Generator Maintenance: Predictive maintenance techniques have been applied to hydropower generators to predict bearing failures and detect abnormal vibrations. These insights help schedule maintenance activities during planned downtime and prevent catastrophic failures.
Conclusion
Predictive maintenance is a game-changer in the renewable energy sector, enabling proactive and targeted maintenance practices. By leveraging data analysis and machine learning, maintenance teams can anticipate equipment failures in renewable energy infrastructure, reduce downtime, extend equipment lifespan, and optimize maintenance planning. The implementation of predictive maintenance requires comprehensive data collection, advanced machine learning models, and integration with existing maintenance workflows. As the renewable energy industry continues to expand, embracing predictive maintenance will become increasingly vital to ensure the efficiency and reliability of renewable energy infrastructure.