Advancements in Solar Power Plant Operations through Machine Learning
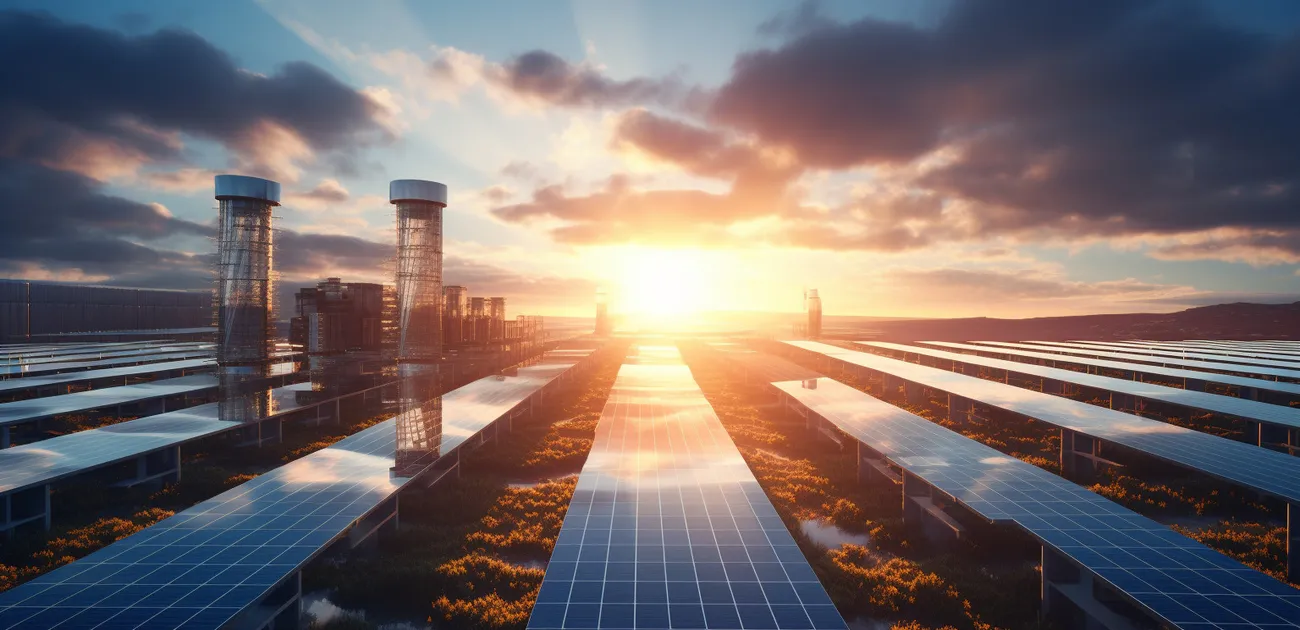
Table of Contents
- Introduction
- Understanding Solar Power Plants
- Challenges in Solar Power Plant Operations
- Role of Machine Learning in Solar Power Plant Operations
- Use Cases of Machine Learning in Solar Power Plants
- Benefits and Future Potential
- Conclusion
Introduction
With the increasing focus on renewable energy sources, solar power plants have gained significant attention. These plants harness energy from the sun and convert it into usable electricity, contributing to a cleaner and more sustainable future. To optimize the operations of solar power plants and enhance their efficiency, the integration of machine learning techniques has emerged as a game-changer. In this blog post, we will delve into the advancements in solar power plant operations through machine learning, exploring the challenges, use cases, and the immense potential for the future.Understanding Solar Power Plants
Before we dive into the role of machine learning, let's first understand the basics of solar power plants. Solar power plants generate electricity by harnessing sunlight through photovoltaic (PV) panels or concentrating solar power (CSP) systems. PV panels directly convert sunlight into electricity using semiconductors, while CSP systems use mirrors or lenses to concentrate sunlight onto a receiver to generate thermal energy that drives a turbine for electricity generation.Challenges in Solar Power Plant Operation
Operating solar power plants efficiently comes with its own set of challenges. Some key challenges include:- Intermittency: Solar power generation is dependent on weather conditions and is subject to intermittent sunlight, which can impact the stability and reliability of the power output.
- Performance Monitoring: Monitoring the performance of individual solar panels, identifying faults or failures, and maintaining optimal output across the entire plant can be a complex task.
- Fault Detection and Diagnostics: Detecting and diagnosing faults in the solar power plant's components, such as inverters, transformers, or cabling, is crucial to ensure smooth operations.
- Maintenance Planning: Timely maintenance is essential to prevent breakdowns and optimize the overall performance of the plant. However, planning and scheduling maintenance activities can be challenging without accurate predictions.
Role of Machine Learning in Solar Power Plant Operations
Machine learning techniques play a vital role in addressing the challenges faced in solar power plant operations. By analyzing large volumes of data collected from various sources within the plant, machine learning models can provide valuable insights and enable proactive decision-making. Here are some ways machine learning is transforming solar power plant operations:1. Predictive Maintenance
Machine learning algorithms can analyze historical data, sensor readings, and other operational parameters to predict the maintenance requirements of solar power plant components. By detecting patterns and anomalies, these models can identify potential failures or performance degradation, allowing plant operators to schedule maintenance activities proactively and minimize downtime.
2. Performance Optimization
Machine learning models can optimize the performance of solar power plants by analyzing real-time and historical data to identify factors affecting efficiency. By considering variables such as weather conditions, panel soiling, and electrical losses, these models can provide recommendations to improve energy output and maximize plant efficiency.
3. Anomaly Detection
Machine learning algorithms can detect anomalies in the operation of solar power plants, helping identify and diagnose issues promptly. By continuously monitoring data streams and comparing them to expected patterns, these models can raise alarms and assist in troubleshooting, reducing the impact of faults and failures.
4. Load Forecasting
Accurate load forecasting is crucial for efficient power grid management. Machine learning techniques can analyze historical data and external factors like weather patterns and public events to predict future electricity demand accurately. Solar power plant operators can leverage this information to optimize their operations, plan energy storage requirements, and ensure a stable power supply.
Use Cases of Machine Learning in Solar Power Plants
Machine learning has already found numerous applications in solar power plants. Some notable use cases include:1. Irradiance Forecasting
By analyzing historical weather data and satellite imagery, machine learning models can forecast solar irradiance levels at specific locations. This information helps plant operators predict energy generation, plan maintenance activities, and optimize the grid integration of solar power.
2. Automated Cleaning and Maintenance
Machine learning can automate the process of solar panel cleaning by analyzing real-time images or videos. By detecting dirt or debris accumulation on panels, these models can schedule cleaning activities, ensuring maximum energy generation and reducing manual labor.
3. Energy Demand Response
Machine learning algorithms can enable solar power plants to respond to fluctuating energy demands in real-time. By predicting demand patterns and adjusting power generation or grid integration accordingly, these models contribute to efficient load management and grid stability.
4. Fault Diagnosis and Prognosis
Machine learning models can analyze sensor data and historical records to diagnose faults in solar power plant components. By identifying patterns and signatures of potential failures, these models enable proactive maintenance, minimizing downtime and repair costs.
Benefits and Future Potential
The integration of machine learning in solar power plant operations offers several benefits and holds tremendous future potential. Some key advantages include: - Improved Plant Efficiency: Machine learning models can optimize the energy output of solar power plants, leading to increased efficiency and enhanced financial returns. - Cost Reduction: Predictive maintenance and fault diagnosis help minimize downtime, reduce repair costs, and optimize the use of resources. - Enhanced Grid Integration: Accurate load forecasting and demand response capabilities contribute to better integration with the power grid, ensuring stability and reliability. - Environmental Impact: By maximizing the energy output and efficiency of solar power plants, machine learning indirectly contributes to reducing carbon emissions and promoting a cleaner environment.Looking ahead, the future potential of machine learning in solar power plant operations is vast. Continued advancements in algorithms, data collection, and sensor technology will further enhance the accuracy and reliability of machine learning models. As solar power continues to play a pivotal role in the global energy transition, the application of machine learning techniques will only become more critical.