Addressing Efficiency Gaps in Biogas Plants through Data-driven Solutions
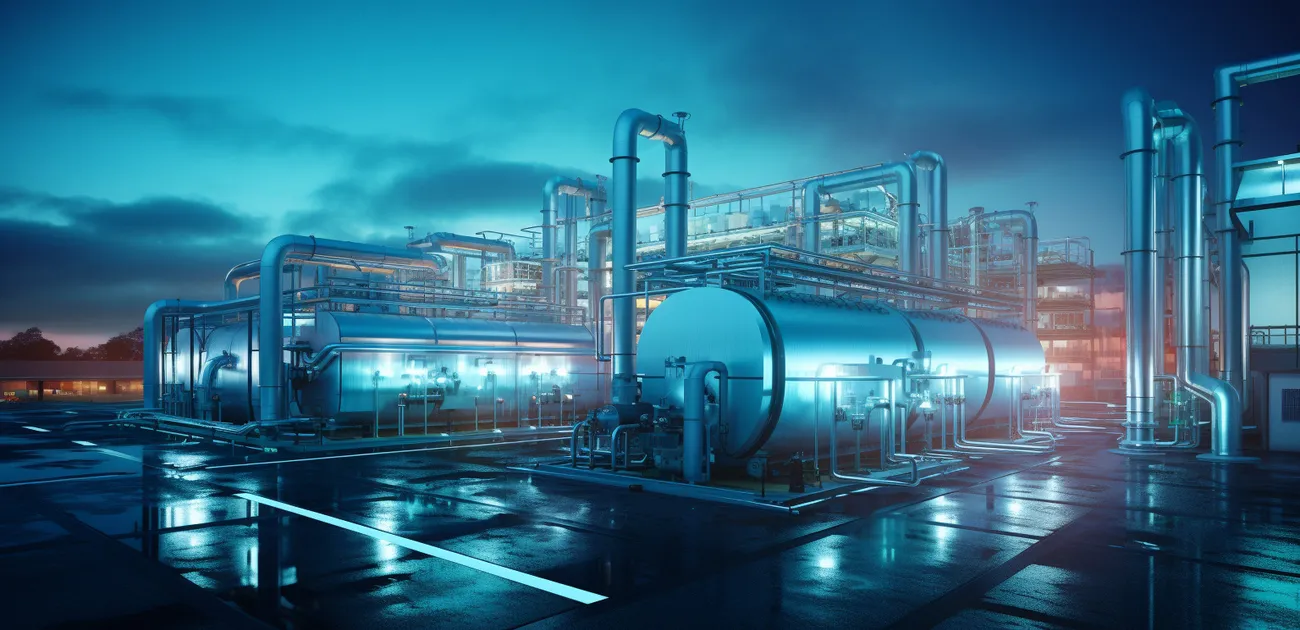
Addressing Efficiency Gaps in Biogas Plants through Data-driven Solutions
Table of Contents
- Introduction
- Understanding Biogas Plants
- Challenges in Biogas Plant Efficiency
- Role of Data-driven Solutions
- Implementing Data-driven Solutions
- Benefits of Data-driven Approaches
- Case Studies
- Conclusion
Introduction
Biogas plants play a vital role in sustainable energy production, converting organic waste materials into valuable renewable energy. However, like any complex system, biogas plants face efficiency gaps that can hinder their performance and output. In this blog post, we will explore how data-driven solutions can help address these gaps and optimize the efficiency of biogas plants.
Understanding Biogas Plants
Before diving into the efficiency gaps, let's briefly understand the workings of a typical biogas plant. Biogas is generated through the anaerobic digestion of organic waste, such as agricultural residues, food waste, and sewage sludge. The process involves breaking down the organic matter by microorganisms in an oxygen-free environment, resulting in the production of biogas, which primarily contains methane (CH4) and carbon dioxide (CO2).
Biogas can be used for various purposes, including electricity generation, heat production, and as a vehicle fuel. However, the efficiency of biogas production can vary due to several factors, leading to suboptimal performance and lower energy yields.
Challenges in Biogas Plant Efficiency
Several challenges contribute to efficiency gaps in biogas plants. Identifying these challenges is crucial to implementing effective data-driven solutions. Let's explore some common factors:
Feedstock Variability
Biogas plants rely on organic waste as feedstock, which can vary in composition and quality. Fluctuations in feedstock properties, such as moisture content, nutrient composition, and pH levels, can impact the overall efficiency of the biogas production process. Analyzing and managing these variations is essential for consistent and optimal plant performance.
Substrate Imbalance
The balance between carbon-rich (C) and nitrogen-rich (N) substrates is crucial for efficient biogas production. Imbalances in substrate ratios can affect the microbial activity during anaerobic digestion, leading to process inefficiencies. Data-driven solutions can help monitor and adjust the feedstock composition to maintain the ideal C:N ratio.
Process Monitoring and Control
Effective process monitoring and control are vital for ensuring optimal plant performance. Traditional methods of manual monitoring and control may not provide real-time insights into crucial parameters such as temperature, pH, and gas composition. Data-driven solutions enable continuous monitoring, analysis, and predictive modeling to identify anomalies, optimize process parameters, and improve overall efficiency.
Digester Performance Analysis
The anaerobic digester is the core component of a biogas plant, where organic waste is broken down by microorganisms. The performance of the digester can be affected by factors such as hydraulic retention time, temperature, and microbial activity. Data-driven analytics can help assess digester performance, identify bottlenecks, and suggest improvements for enhanced biogas production.
Role of Data-driven Solutions
Data-driven solutions offer valuable insights and opportunities for optimizing biogas plant efficiency. By leveraging data analytics, machine learning, and automation, these solutions empower operators and plant managers to make informed decisions and take proactive actions. Here are some key areas where data-driven solutions prove beneficial:
Feedstock Analysis and Management
Accurate and real-time analysis of feedstock composition and quality can help optimize the digestion process. Data-driven solutions enable continuous monitoring of feedstock properties, such as moisture content, organic matter content, and nutrient composition. By analyzing this data, operators can adjust the feedstock mixture, implement pretreatment methods, or make informed decisions regarding alternative feedstocks.
Real-time Process Monitoring
Data-driven solutions enable real-time monitoring of critical parameters such as temperature, pH, and gas composition within the digester. Advanced sensors and data analytics platforms provide a comprehensive view of the plant's performance. Operators can detect deviations from optimal conditions, identify potential issues, and take corrective actions promptly.
Predictive Analytics and Maintenance
Data-driven models can predict potential equipment failures or maintenance needs based on historical data and real-time sensor inputs. By implementing predictive maintenance strategies, operators can minimize downtime, optimize equipment performance, and reduce maintenance costs. This proactive approach ensures smooth operation and avoids unexpected disruptions.
Optimization of Plant Parameters
Data-driven solutions can analyze vast amounts of data to identify patterns, correlations, and optimization opportunities. By analyzing historical performance data and conducting simulations, operators can fine-tune process parameters such as hydraulic retention time, temperature, and substrate composition. This optimization helps achieve maximum biogas production and energy efficiency.
Implementing Data-driven Solutions
Implementing data-driven solutions in biogas plants requires a systematic approach. Here are the key steps involved:
Data Collection and Integration
Collecting data from various sources within the biogas plant is the first step. This may involve installing sensors, data loggers, or integrating with existing process control systems. The data collected should include feedstock properties, process parameters, gas composition, and other relevant variables.
Data Storage and Management
An efficient data storage and management system is essential to handle the large volumes of data generated by biogas plants. Cloud-based platforms or local servers can be used to store and process the data securely. It's crucial to ensure data integrity, backup procedures, and compliance with data protection regulations.
Data Analysis and Visualization
Data analytics tools and algorithms are used to process and analyze the collected data. Statistical analysis, machine learning, and visualization techniques help identify trends, patterns, and correlations. Interactive dashboards and reports enable easy interpretation and decision-making based on the data insights.
Integration with Automation and Control Systems
To enable real-time monitoring and control, data-driven solutions should be integrated with automation and control systems. This integration allows seamless communication between the data analytics platform and the plant's control infrastructure. Automated alerts, notifications, and control actions can be triggered based on predefined rules or anomalies detected by the data-driven system.
Benefits of Data-driven Approaches
Adopting data-driven approaches in biogas plants offers numerous benefits:
Improved Efficiency and Energy Yields
By addressing efficiency gaps and optimizing process parameters, data-driven solutions enhance the overall efficiency of biogas plants. This results in increased energy yields from the same feedstock, maximizing the renewable energy output.
Reduced Operating Costs
Efficient monitoring, predictive maintenance, and optimized process parameters help reduce operating costs associated with energy consumption, maintenance, and waste management. Data-driven solutions enable proactive decision-making, minimizing downtime and resource wastage.
Enhanced Environmental Sustainability
Biogas plants contribute to environmental sustainability by diverting organic waste from landfills and reducing greenhouse gas emissions. Data-driven solutions further enhance this sustainability by optimizing the biogas production process, reducing resource consumption, and improving waste management practices.
Scalability and Replicability
Data-driven solutions can be scaled and replicated across multiple biogas plants. Lessons learned from one plant can be applied to others, accelerating efficiency improvements and standardizing best practices.
Case Studies
Case Study 1: XYZ Biogas Plant
XYZ Biogas Plant implemented a data-driven solution to address efficiency gaps. By continuously monitoring feedstock properties, process parameters, and gas composition, the plant optimized the feedstock mixture, adjusted process parameters, and fine-tuned digester performance. As a result, the plant achieved a 20% increase in biogas production and reduced operating costs by 15%.
Case Study 2: ABC Biogas Plant
ABC Biogas Plant deployed a data analytics platform to analyze historical data and predict maintenance needs. By proactively addressing equipment failures and optimizing maintenance schedules, the plant reduced downtime by 25% and saved 10% in maintenance costs.
Conclusion
Addressing efficiency gaps in biogas plants through data-driven solutions offers immense potential for optimizing renewable energy production. By leveraging advanced analytics, real-time monitoring, and predictive modeling, biogas plant operators can improve efficiency, reduce operating costs, and contribute to environmental sustainability. As the demand for renewable energy continues to grow, embracing data-driven approaches becomes paramount for maximizing the potential of biogas plants in the transition towards a greener future.